By Jill Hubbard Bowman
The main goal of this AI Law Maze Map blog is to help you navigate the twists and turns of AI law. But to get on the path, you must understand some AI terminology and how AI systems are created. After briefly explaining some basics in this Key Terms series, including descriptions of AI models in part 3 and more about data in part 4, this blog will discuss the complex legal issues at every turn. A full Key Terms resource guide including all the definitions in the series will be provided at the end of the series for your convenience.
The reason people who care about legal compliance should know more about the types of machine learning, in part, is because the type and location of the data used in the learning process may vary the type and level of legal risk. For example, federated learning is a great way to minimize privacy risk to very sensitive personal information, like healthcare data. Supervised learning can add legal complexity in contracts when humans are creating databases of factual information in multiple countries without database rights and the need to keep the information as a trade secret is high. Adversarial learning may hijack a model and result in legal liability for a product owner.
Below are some definitions for machine learning:
Machine Learning
Machine learning is a sub-field of AI computer science studying how machines can learn by themselves through data and experience, somewhat like humans. Generally, machine learning refers to a group of techniques used to create and train AI models. Specifically, machine learning is a type of data processing using training data sets and statistical techniques to identify patterns without explicit rules. Machine learning techniques are useful for making predictions, classifying objects, processing natural language, and recognizing speech.
There are many types of machine learning used to train models: federated, supervised, unsupervised, and semi-supervised. A malicious type of learning is called adversarial learning.
Federated Learning
Federated learning is a machine learning process where the model is trained on localized data. Only the updates to the model are sent to another location, not the data itself, for aggregation with other versions of the models until the model if fully trained. This process allows for better privacy and security for sensitive data like health care information.
Supervised Learning
Supervised learning is a machine learning process where a model learns to make accurate predictions after “seeing” human-labeled data. For example, to help an object recognition model correctly identify a cat, the model is not explicitly told to look for a small, furry creature with pointed ears, whiskers, and a long tail. Rather, the model is shown many images of cats labeled as cats. Then, without human intervention, the model will figure out the characteristics of a cat. As it learns from the known inputs, the model automatically updates its weights through the array to improve its statistical calculations and the probability it will predict that the image of a cat is a cat – even a hairless sphynx cat.
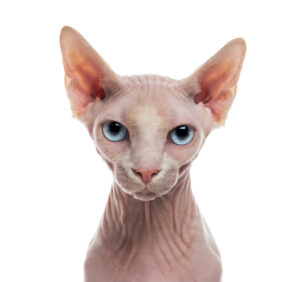
Close-up of a Sphynx
Unsupervised Learning
Unsupervised learning is the machine learning process where a model learns to infer relationships from unlabeled data. For example, a large language model learns how to predict the next word in a sentence from reviewing massive amounts of text and learning about the relationships and patterns in the text.
Semi-supervised Learning
Semi-supervised learning is a hybrid machine learning approach for training a model. Labeled data sets are expensive and often difficult to obtain. With semi-supervised learning both unlabeled and labeled data are used, saving time and money, while still achieving accuracy. This is important for models trained on massive amounts of data like large language models.
Adversarial Learning
Adversarial learning is a type of machine learning technique where deceptive inputs are used to trick the machine learning model into providing inaccurate outputs. This model manipulation is a type of attack and jeopardizes the safety and security of the system.
In the next part of the Key Terms series, I will explain more about AI models. Understanding those nuances are critical to the legal controversies about application of the law to the core component of AI systems.
Jill Hubbard Bowman is an attorney specializing in AI Intellectual Property law. From the first wave of the internet to the new wave of artificial intelligence, Jill has helped high technology companies navigate cutting edge legal issues for over twenty-five years.